In the realm of artificial intelligence (AI), spiking neural networks (SNNs) have emerged as a groundbreaking technology, mimicking the functionality of the human brain in processing information. With their unique approach to computing, SNNs hold the potential to revolutionize various domains, from neuroscience to robotics and beyond.
Spiking neural networks (SNNs) are a type of artificial neural network (ANN) that is inspired by the way biological neurons communicate with each other. Unlike traditional ANNs, which use continuous values to represent information, SNNs use spikes, or brief pulses of activity, to encode and transmit information. This makes SNNs more biologically realistic and potentially more energy-efficient than traditional ANNs.
Understanding Spiking Neural Networks
At the core of spiking neural networks lies the concept of neurons communicating through discrete spikes or pulses of activity, akin to the firing of neurons in the human brain. Unlike traditional artificial neural networks (ANNs) that operate based on continuous values, SNNs rely on the timing and frequency of these spikes to encode and process information. This spike-based communication enables SNNs to efficiently represent and process complex data patterns, making them highly suitable for tasks requiring real-time processing and event-based computation.
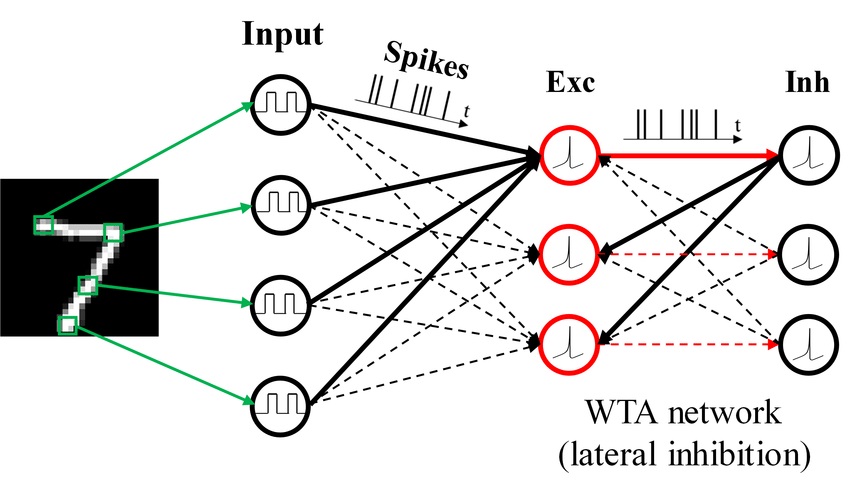
Applications of Spiking Neural Networks
1. Neuromorphic Computing
One of the key applications of SNNs is in the field of neuromorphic computing, where researchers aim to develop hardware and software systems that mimic the structure and function of the human brain. SNNs offer significant advantages in this domain due to their low power consumption, parallel processing capabilities, and ability to handle asynchronous input signals. These attributes make SNNs ideal for applications such as pattern recognition, sensor data processing, and adaptive control systems.
2. Brain-Computer Interfaces (BCIs)
In the realm of neuroscience and healthcare, spiking neural networks play a vital role in the development of brain-computer interfaces (BCIs). By leveraging the brain’s natural spiking activity, researchers can decode neural signals and translate them into commands for controlling external devices or prosthetics. SNN-based BCIs hold promise for restoring mobility and communication abilities to individuals with paralysis or neurological disorders, offering new avenues for assisted living and rehabilitation.
3. Event-based Vision Sensors
Event-based vision sensors, inspired by the human retina, operate on the principle of detecting and transmitting visual information in the form of asynchronous spikes. Spiking neural networks are well-suited for processing data from these sensors, enabling efficient object recognition, motion tracking, and scene analysis in real-time. The event-driven nature of SNNs allows for rapid processing of visual stimuli while conserving computational resources, making them ideal for applications in autonomous vehicles, surveillance systems, and robotics.
4. Cognitive Computing
In the domain of artificial intelligence, cognitive computing systems aim to emulate human-like reasoning and decision-making capabilities. Spiking neural networks offer a promising framework for building such systems by simulating the intricate dynamics of neuronal activity and synaptic plasticity. By incorporating principles of spiking neurons and spike-timing-dependent plasticity (STDP), SNNs can learn from temporal patterns in data, adapt to changing environments, and exhibit advanced cognitive functions such as learning, memory, and inference.
Future Outlook and Challenges
Despite their immense potential, spiking neural networks still face several challenges that must be addressed for widespread adoption and advancement. These include developing efficient training algorithms, optimizing hardware implementations, and bridging the gap between theoretical models and practical applications. However, with ongoing research efforts and collaborations across disciplines, the future looks promising for SNNs to unlock new frontiers in artificial intelligence and reshape the way we perceive and interact with technology.
Here are some of the key features of SNNs:
- Neurons: SNNs are made up of artificial neurons that model the behavior of biological neurons. These neurons have a state, which is typically represented by their membrane potential. When the membrane potential reaches a certain threshold, the neuron fires a spike.
- Synapses: The connections between neurons in an SNN are called synapses. Synapses can be excitatory, which means they increase the membrane potential of the postsynaptic neuron, or inhibitory, which means they decrease the membrane potential of the postsynaptic neuron.
- Timing: The timing of spikes is important in SNNs. The order in which spikes arrive at a neuron, as well as the time between spikes, can all affect the neuron’s output.
SNNs are still under development, but they have the potential to revolutionize the field of artificial intelligence. They have a number of advantages over traditional ANNs, including:
- Biological realism: SNNs are more biologically realistic than traditional ANNs, which could make them better suited for understanding and modeling the brain.
- Energy efficiency: SNNs are potentially more energy-efficient than traditional ANNs, as they only use energy when they are spiking.
- Temporal processing: SNNs are well-suited for processing temporal information, such as speech and video.
However, there are also some challenges associated with SNNs, including:
- Training: Training SNNs can be more difficult than training traditional ANNs, as the non-differentiable nature of spiking signals makes it difficult to use backpropagation.
- Hardware: SNNs require specialized hardware to run efficiently, which is not yet widely available.
Conclusion
In conclusion, spiking neural networks represent a paradigm shift in the field of artificial intelligence, offering a biologically-inspired approach to information processing that holds tremendous promise across various domains. From neuromorphic computing to brain-computer interfaces and beyond, SNNs are poised to drive innovation and transform industries in ways previously unimaginable. By delving deeper into the principles and applications of spiking neural networks, we pave the way for a future where intelligent systems seamlessly integrate with our lives, enhancing efficiency, and enriching human experiences.